The Power of an Open Source Image Annotation Tool
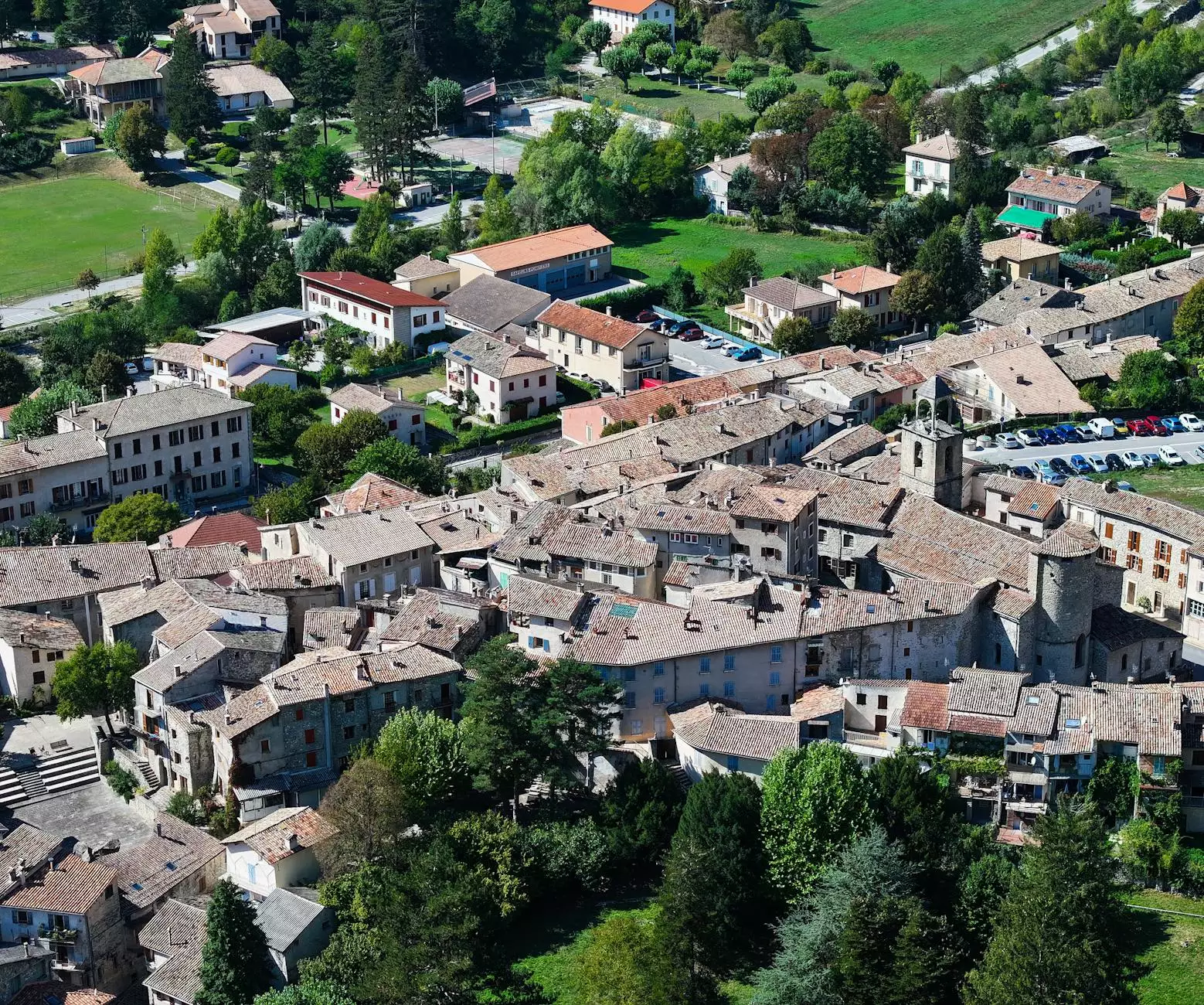
In today's data-driven world, the significance of effective data annotation cannot be overstated. As businesses and organizations continue to harness the potential of artificial intelligence (AI) and machine learning (ML), the demand for accurate data labeling is skyrocketing. Among the various methods for data annotation, using an image annotation tool open source has emerged as a powerful solution that not only streamlines the annotation process but also makes it accessible to all.
Understanding Image Annotation
Image annotation involves labeling images to provide more context and meaning, which is crucial for AI and ML systems to learn and make decisions. It includes various tasks such as bounding box annotation, semantic segmentation, and image classification. These annotations serve as training data for models, enabling them to recognize patterns and make predictions in real-world applications.
Why Choose an Open Source Image Annotation Tool?
Utilizing an image annotation tool open source brings numerous advantages:
- Cost-Effective: Open source tools are generally free to use, which significantly reduces operational costs for businesses.
- Customizable: These tools can be modified to suit specific project requirements, allowing for a tailored experience.
- Community Support: Open source projects typically have vibrant communities that contribute to improvements, bug fixes, and support. This can enhance the overall functionality and reliability of the tool.
- Transparency: Being open source means that users can inspect the code, ensuring that there are no hidden functionalities or costs.
- Ease of Integration: Many open source annotation tools can easily integrate with other software platforms, creating a seamless workflow.
Key Features of an Effective Image Annotation Tool
When looking for an image annotation tool open source, it is crucial to consider specific features that enhance usability and efficiency. Here are some of the fundamental features to look out for:
- User-Friendly Interface: A clean, intuitive interface allows users to focus on the tasks at hand without the hassle of figuring out complicated functionalities.
- Support for Multiple Annotation Types: A good tool should support various annotation types including bounding boxes, polygons, and keypoints.
- Collaboration Tools: Facilitating teamwork through shared projects and centralized asset management can significantly enhance productivity.
- Data Export Options: The ability to export data in different formats (like COCO, PASCAL VOC, or CSV) caters to various machine learning frameworks.
- Integration Capabilities: Seamless integration with other platforms like TensorFlow, PyTorch, or your existing data pipeline is essential.
Popular Open Source Image Annotation Tools
There are several reputable image annotation tool open source options available. Here are some worth considering:
1. LabelImg
LabelImg is a widely-used open source image annotation tool that supports bounding box annotations. It allows users to create rectangular boxes around objects in images, making it perfect for object detection tasks.
2. VGG Image Annotator (VIA)
The VGG Image Annotator is a versatile image annotation tool that supports various annotation forms such as regions, polygons, and points. Its lightweight nature allows it to run in a web browser, making it accessible from any device.
3. CVAT
Developed by Intel, CVAT (Computer Vision Annotation Tool) is designed for professional users and supports a wide range of annotation formats. Its collaborative capabilities enable teams to work together on projects efficiently.
4. RectLabel
RectLabel focuses on ease of use, allowing users to annotate images quickly with bounding boxes and polygons. It is especially popular among those working with TensorFlow and other machine learning frameworks.
How to Choose the Right Image Annotation Tool
Choosing the right image annotation tool open source for your business involves a few crucial steps:
- Assess Your Needs: Understand the specific types of annotations you need and the scale of your projects. Will you require the ability to annotate video as well?
- Evaluate the Learning Curve: Consider how easy it is for your team to learn and use the tool. A steep learning curve can hinder productivity.
- Test with Demos: Many open source tools offer demo versions. Testing with real images helps you understand the capabilities and limitations.
- Check for Community Support: A solid community can be invaluable for troubleshooting issues and gaining insights on best practices.
- Consider Future Scalability: Ensure that the tool can scale with your company's growth and adapt to future needs.
The Role of Image Annotation in AI and Machine Learning
The accuracy of machine learning and AI models significantly depends on the quality of data they are trained on. High-quality annotations allow models to learn correctly and make accurate predictions. Here are some reasons why image annotation is critical:
- Enhanced Model Accuracy: Well-annotated datasets lead to better-trained models, resulting in improved accuracy in prediction tasks.
- Reduces Model Bias: Diverse and accurately labeled datasets help reduce bias, making models fairer and more reliable.
- Support for Different Applications: From autonomous vehicles to healthcare diagnostics, accurate image annotation serves various industries and applications.
Integrating Image Annotation into Your Workflows
To maximize the benefits of an image annotation tool open source, it is essential to integrate it efficiently into your existing workflows:
- Establish Clear Guidelines: Providing clear annotation guidelines ensures consistency across the team, leading to better dataset quality.
- Regular Training: Conduct regular training sessions based on the annotation tasks to improve team competency.
- Monitor Progress: Using project management tools can help track the progress and quality of annotations across team members.
- Adopt a Review Process: Implementing a review process for annotations helps catch errors early, ensuring data integrity.
The Future of Image Annotation Tools
The future of image annotation tools looks promising. With advancements in AI, we can expect the emergence of semi-automated and fully automated annotation tools, which will significantly reduce the manual overhead that currently exists. Here are some trends to watch:
- AI-Assisted Annotation: New tools are incorporating AI algorithms that can suggest or even create annotations automatically, enhancing efficiency.
- Collaborative Annotations: Tools that facilitate real-time collaboration among team members are becoming increasingly essential.
- Cloud-Based Solutions: As teams become more remote, cloud-based annotation tools will facilitate access from anywhere.
- Enhanced Security Features: With the growing concern over data privacy, new tools will focus on ensuring secure data handling and storage.
Conclusion
In conclusion, image annotation tools open source have revolutionized the way businesses handle data annotation. By choosing the right tool and implementing best practices, organizations can enhance their data labeling processes, leading to improved outcomes in AI and ML projects. The flexibility, cost-effectiveness, and collaborative features of these tools make them an invaluable asset for any organization aiming to harness the power of data.
For more information about open source image annotation tools and their role in enhancing data practices, visit keylabs.ai.