Unlocking the Power of Machine Learning Labeling Tools
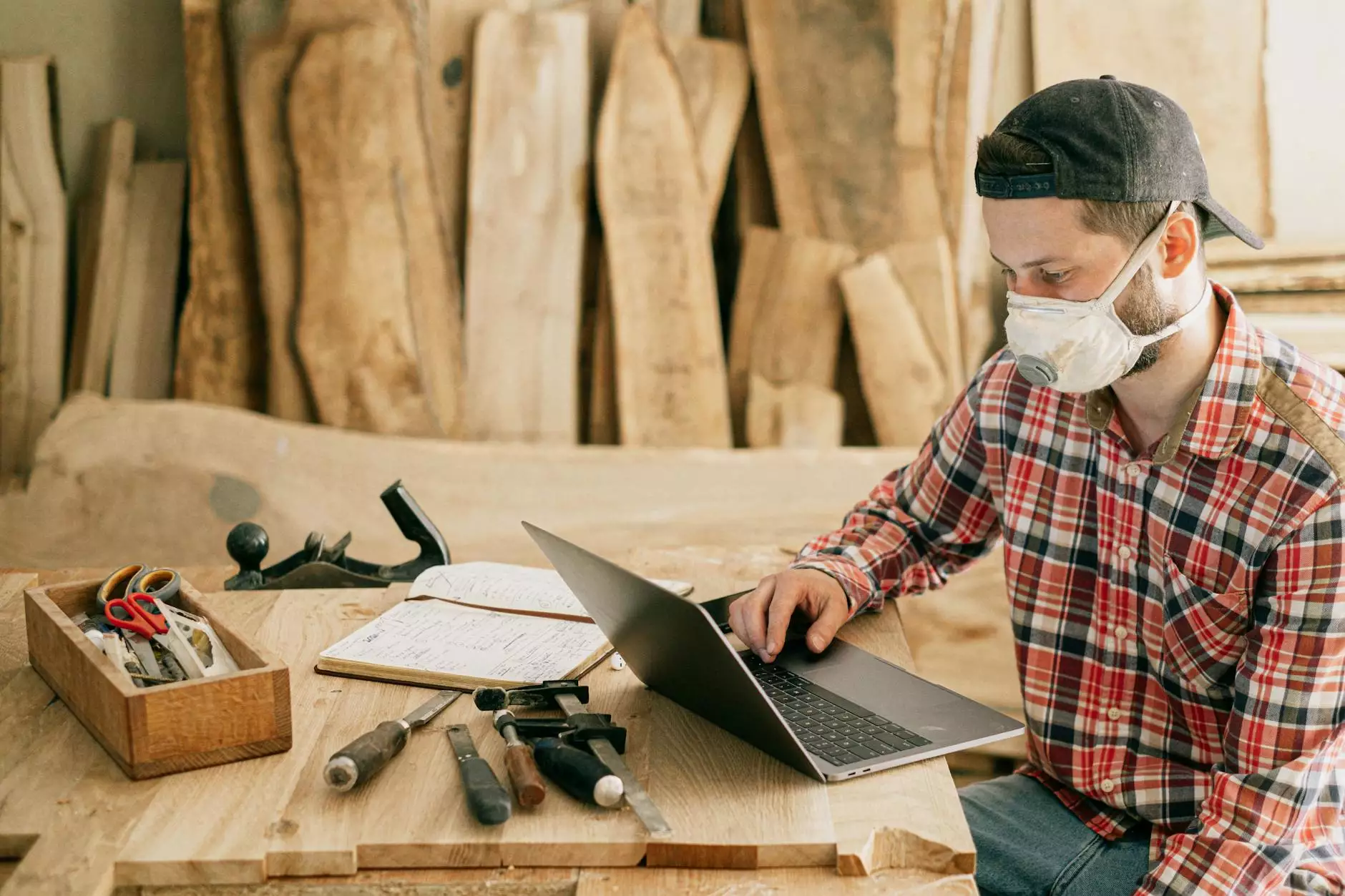
In the ever-evolving landscape of artificial intelligence, the demand for high-quality data has never been more crucial. The success of machine learning models heavily depends on the data fed into them, and that’s where machine learning labeling tools come into play. These tools play an essential role in creating accurate datasets that enhance the learning capabilities of AI algorithms. In this detailed guide, we will explore the diverse features and benefits of machine learning labeling tools, focusing on how these tools can streamline your data annotation processes.
What is a Machine Learning Labeling Tool?
A machine learning labeling tool is a software solution designed to facilitate the data annotation process. Data annotation involves assigning metadata, tags, or labels to various data types—be it images, text, audio, or video—making it easier for machine learning algorithms to understand and learn patterns. This process is critical because the accuracy of a model's predictions largely hinges on the quality of the training data it receives.
Why is Data Annotation Important?
The primary purpose of data annotation is to create high-quality, labeled datasets that serve as a foundation for training machine learning models. The significance of this process can be highlighted through the following points:
- Improved Model Accuracy: Labeled data ensures that algorithms can learn effectively, leading to higher prediction accuracy.
- Enhanced Understanding: Annotated data helps algorithms discern complex patterns, which is vital for tasks such as image recognition and natural language processing.
- Diverse Applications: From autonomous vehicles to healthcare diagnostics, machine learning labeling tools support a wide range of industries that require accurate data classification.
Key Features of Quality Machine Learning Labeling Tools
Choosing the right data annotation tool is essential for optimizing the data labeling process. Here are some key features to look out for:
1. User-Friendly Interface
A user-friendly interface is critical for both novice and experienced users. An intuitive design allows users to perform annotation tasks efficiently without extensive training.
2. Support for Multiple Data Formats
The best machine learning labeling tools support various data formats, including text, images, audio, and video. This flexibility enables development teams to work with different data types seamlessly.
3. Scalability
As projects grow, so do the data requirements. A scalable labeling tool can handle increasing amounts of data without sacrificing performance.
4. Collaboration Features
Effective collaboration features allow multiple team members to work together on annotation projects, ensuring that everyone is aligned and reducing errors.
5. Flexibility and Customization
The ability to customize labels and create specific annotation workflows is vital, as it allows the tool to adapt to unique business needs and objectives.
6. Integration Capabilities
Integration with existing tools and machine learning frameworks simplifies the workflow and enhances productivity. Look for tools that offer plugins or API support for easy connectivity.
Popular Types of Data Annotation
Machine learning labeling tools can be utilized for various types of data annotation. Below are some prevalent forms:
Image Annotation
Image annotation is critical for computer vision tasks. Common techniques include:
- Bounding Boxes: Enclosing objects within a rectangular box.
- Segmentation: Classifying each pixel in an image to identify different objects.
- Keypoint Annotation: Marking specific points such as joints in a body for pose estimation.
Text Annotation
Text annotation is essential for natural language processing tasks. It can include:
- Entity Recognition: Identifying entities like names, dates, and locations.
- Sentiment Analysis: Tagging text segments by sentiment (positive, negative, neutral).
- Intent Classification: Defining the goal behind a user's query.
Audio Annotation
In audio annotation, the focus is on transcription and sound labeling. Techniques include:
- Speech Transcription: Converting spoken words into written text.
- Sound Classification: Tagging different sounds in audio files.
Top Machine Learning Labeling Tools in the Market
Several tools are making significant strides in the field of data annotation. Below are some top alternatives worth considering:
1. Keylabs.ai
At the forefront of data annotation is Keylabs.ai. This powerful data annotation platform enables seamless labeling of vast datasets efficiently. Its comprehensive set of features, including collaborative tools and multi-format support, makes it a preferred choice for AI developers.
2. Labelbox
Labelbox is another robust platform that focuses on accelerating the data labeling process. Its user-friendly interface promotes customization and integrates well with various machine learning workflows.
3. VGG Image Annotator (VIA)
VIA is an open-source project that caters to those who seek a cost-effective solution for image and video annotation. It's a lightweight platform that facilitates quick labeling tasks.
4. Supervisely
Supervisely offers a comprehensive suite of tools for data annotation, including capabilities for both image and video projects. Its advanced features allow for high-quality annotations that drive better machine learning outcomes.
Challenges in Data Annotation
While data annotation is essential, it is not without its challenges. Some common issues include:
Quality Control
Ensuring the consistency and accuracy of annotations can be difficult, especially with large datasets. Inaccurate labeling can lead to poor model performance.
Time Consumption
Manual data annotation can be a labor-intensive process, consuming valuable time that could be spent on model training and improvement.
Scalability Issues
As datasets grow, so does the challenge of keeping up with the annotation demands. Scaling the labeling process efficiently requires proper tools and frameworks.
Best Practices for Data Annotation
To maximize the effectiveness of your machine learning labeling tools, consider implementing the following best practices:
1. Define Clear Guidelines
Establishing clear annotation guidelines helps annotators understand expectations and reduces variability in the labeling process.
2. Use Pre-Labeled Data for Training
Employ pre-labeled datasets to train your annotation team. This can serve as a reference, aiding them in producing more accurate annotations.
3. Implement Frequent Reviews
Regularly review annotated data to catch errors early. This feedback loop helps maintain quality and reliability in your datasets.
4. Leverage Automation
Consider using semi-automated labeling tools that utilize AI to assist with the annotation process. This can significantly reduce time and effort.
The Future of Machine Learning Labeling Tools
As artificial intelligence continues to evolve, machine learning labeling tools are also poised for growth. We can expect innovations such as:
- Increased Automation: AI-driven automation will play a more significant role in labeling tasks, reducing reliance on manual input.
- Advanced Collaboration Features: Enhanced collaborative tools will facilitate better teamwork among data scientists and annotators.
- Integration with Advanced ML Models: Future tools will likely integrate better with advanced machine learning models, enabling real-time feedback loops for continuous improvement.
Conclusion
Investing in the right machine learning labeling tool is essential for the success of your AI projects. By ensuring high-quality data annotation, businesses can enhance the performance of their machine learning models while saving time and resources. With platforms like Keylabs.ai, organizations can navigate the complexities of data labeling with ease and efficiency. The future is bright for machine learning labeling tools, and those who harness their capabilities will stay ahead in the competitive landscape of AI.